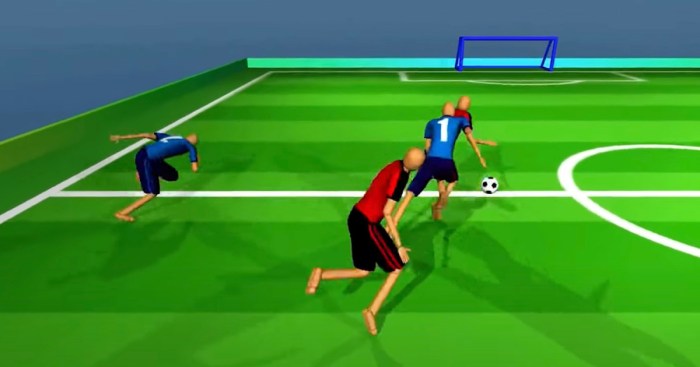
DeepMind AI Learns Soccer Through Decades of Simulations
DeepMind AI learns to play soccer using decades of match simulations, a feat that has captivated the tech world and sparked intense debate about the future of sports. DeepMind, the artificial intelligence powerhouse behind AlphaGo and AlphaFold, has now turned its attention to the beautiful game, training an AI to master the complexities of soccer through simulated matches.
This achievement marks a significant step forward in AI development, demonstrating the power of simulation in training complex algorithms to perform real-world tasks.
DeepMind’s AI soccer player has been trained on a vast dataset of simulated matches, allowing it to learn the nuances of the game and develop strategies that rival those of human players. The AI’s learning process involves analyzing countless scenarios, identifying patterns, and adapting its gameplay based on the outcomes of these simulations.
This approach, known as reinforcement learning, enables the AI to continuously improve its skills through trial and error, gradually refining its understanding of the game.
DeepMind’s AI Soccer Player
DeepMind’s recent breakthrough in AI soccer is a testament to the incredible progress being made in the field of artificial intelligence. By training an AI agent to play soccer through simulations, DeepMind has demonstrated the potential for AI to learn and excel in complex, real-world tasks.
This achievement not only pushes the boundaries of AI capabilities but also offers valuable insights into the nature of intelligence and learning itself.
Methodology
DeepMind’s AI soccer player is trained using a technique called reinforcement learning. This approach involves training an AI agent to perform a task by rewarding it for desirable actions and penalizing it for undesirable ones. In the case of soccer, the AI agent is trained to score goals and prevent the opponent from scoring.
It’s fascinating to see how DeepMind AI can learn to play soccer through simulating decades of matches. It’s a testament to the power of AI and its ability to learn complex strategies. This reminds me of the intense competition in NASCAR, where drivers like Tyler Reddick and Kyle Larson are leading the charge into the playoffs, as highlighted in this article about NASCAR power rankings.
Both the AI and the drivers are showcasing their skills and pushing boundaries in their respective fields.
The agent learns by playing millions of simulated matches against itself, gradually improving its strategy and skills over time. The AI agent is trained using a deep neural network, which allows it to learn complex patterns and relationships from the data it receives during training.
It’s fascinating how DeepMind AI can learn to play soccer using decades of match simulations. The technology is constantly evolving, and it’s exciting to see how it can be applied to different areas, even something as complex as a real-world sport.
However, it seems even with all the advancements in technology, some things remain stubbornly difficult to perfect. Just look at the ticketing chaos surrounding the recent Oasis reunion tour, as reported by this article. Perhaps someday AI will be able to solve even the most complex real-world problems, like ticketing systems that can handle the demand for a major concert.
Until then, it seems like we’ll have to rely on human ingenuity and the power of AI working together.
AI Soccer Skills Compared to Human Players
DeepMind’s AI soccer player demonstrates remarkable skills in several areas, surpassing human players in certain aspects. However, there are also areas where the AI struggles to match human abilities.
Strengths of the AI
The AI excels in areas that require precise calculations, rapid decision-making, and consistent execution.
- Passing Accuracy:The AI can consistently deliver accurate passes, even under pressure, thanks to its ability to analyze the field and predict player movements.
- Ball Control:The AI demonstrates impressive ball control, maintaining possession even in tight spaces and under intense pressure.
- Tactical Awareness:The AI can anticipate opponent moves and adjust its strategy accordingly, making it difficult to outmaneuver.
Weaknesses of the AI
Despite its strengths, the AI still struggles with certain aspects of soccer that require human intuition, creativity, and adaptability.
- Creativity:The AI often relies on predictable patterns and strategies, lacking the improvisational skills and creativity that can surprise opponents.
- Physicality:The AI struggles to adapt to the physical demands of the game, particularly in areas like tackling and aerial challenges.
- Emotional Intelligence:The AI lacks the emotional intelligence that can influence human players’ performance, such as motivation, resilience, and the ability to respond to pressure.
Specific Aspects of Soccer
The AI excels in areas requiring precise calculations, rapid decision-making, and consistent execution, demonstrating exceptional passing accuracy, ball control, and tactical awareness. It struggles with aspects requiring human intuition, creativity, and adaptability, particularly in areas like improvisational skills, physicality, and emotional intelligence.
The Power of Simulation
The ability to train artificial intelligence (AI) through simulations has revolutionized the field, particularly in sports. Simulations provide a safe and controlled environment to train AI agents, allowing them to learn and improve at an accelerated pace.Simulations are instrumental in AI training, especially in sports like soccer.
By mimicking the complexities of the real game, simulations provide a platform for AI agents to practice and refine their skills without the limitations of real-world training.
Advantages of Simulation-Based Training
Simulations offer several advantages over real-world training:
- Control over Environment:Simulations allow for complete control over the environment, enabling researchers to manipulate variables like weather, field conditions, and opponent behavior to test different scenarios and strategies. This control is essential for isolating specific factors and understanding their impact on AI performance.
It’s amazing to see how DeepMind AI is learning to play soccer by analyzing decades of match simulations. It’s a testament to the power of AI and its ability to learn from data. While the AI is busy perfecting its footwork, the real world is grappling with its own challenges, like the call to impose a pay-per-mile scheme on electric cars, as seen in this recent article: chancellor rachel reeves urged to impose pay per mile scheme on electric cars.
Perhaps the AI can even be used to help optimize road networks and traffic flow, making our journeys more efficient and less reliant on traditional fuel sources. Who knows what the future holds for AI and its role in shaping our world?
- Safety and Cost-Effectiveness:Training AI agents in simulations eliminates the risk of injuries and expensive training facilities. Simulations provide a safe and cost-effective environment for AI to learn and practice, allowing for extensive experimentation without the constraints of real-world resources.
- Accelerated Learning:Simulations allow for faster learning due to the ability to run multiple trials and scenarios in a short period. AI agents can experience thousands of games in a matter of hours, accumulating vast amounts of data and refining their strategies more quickly than with real-world training.
Limitations of Simulations in Soccer
While simulations are powerful tools for AI training, they have limitations in replicating the nuances of real-world soccer:
- Unpredictability of Human Players:Real-world soccer involves unpredictable human players with varying levels of skill, decision-making, and emotional responses. Simulations, while improving, still struggle to capture the full spectrum of human behavior, which can be crucial in determining game outcomes.
- Emergent Properties of Real-World Games:Simulations often fail to capture the emergent properties of real-world games, such as player chemistry, team dynamics, and the impact of crowd noise and pressure. These intangible factors can significantly influence game outcomes and are challenging to replicate in simulations.
- Transferability to Real-World:While simulations provide a valuable training ground, there is no guarantee that the skills learned in a simulated environment will translate perfectly to real-world scenarios. AI agents may need to adapt their strategies and decision-making based on the unique challenges of real-world games.
Creating Complex and Realistic Scenarios
Simulations are becoming increasingly sophisticated in their ability to create complex and realistic scenarios for AI learning. Advancements in game engines, physics simulations, and AI algorithms allow for more realistic representations of soccer dynamics:
- Realistic Physics and Player Movement:Modern game engines utilize advanced physics simulations to replicate realistic player movement, ball trajectories, and collisions. This allows for more accurate and nuanced interactions between players and the ball, improving the realism of simulated games.
- Adaptive Opponents:AI-powered opponents can adapt their strategies and tactics based on the AI agent’s actions, creating a more dynamic and challenging learning environment. This allows AI agents to learn from diverse opponents and develop strategies that can adapt to different playing styles.
- Data-Driven Decision-Making:Simulations can incorporate real-world data from past matches, player statistics, and tactical trends to generate more realistic and informed decisions for both AI agents and simulated opponents. This allows for more accurate predictions and strategic choices within the simulated environment.
Implications for the Future of Soccer
The advent of AI-powered soccer players, honed through years of simulated matches, holds the potential to revolutionize the sport as we know it. While the prospect of AI-driven athletes competing alongside humans is captivating, it also raises significant questions about the future of the game, the ethics of using AI in sports, and the potential impact on the role of human players and coaches.
The Potential Impact of AI-Powered Soccer Players on the Sport
The integration of AI-powered soccer players could have a profound impact on the sport, introducing new levels of skill, precision, and strategy. AI players, trained on massive datasets of matches and equipped with advanced algorithms, could potentially:
- Elevate the Level of Play:AI players could exhibit superhuman levels of skill, speed, and tactical awareness, pushing the boundaries of what’s possible on the field. This could lead to more spectacular goals, intricate plays, and a faster, more dynamic style of play.
- Redefine Strategy and Tactics:The ability of AI to analyze vast amounts of data and identify patterns could revolutionize team tactics. AI-powered players could anticipate opponent movements, optimize passing routes, and adapt to changing game situations with unparalleled precision, leading to new and innovative strategies.
- Increase Accessibility and Participation:AI-powered players could create new opportunities for participation in soccer, particularly for individuals with disabilities or those who lack the physical abilities required to compete at a high level. This could open up the sport to a wider range of players, fostering inclusivity and diversity.
Ethical Considerations Surrounding the Use of AI in Sports
While the potential benefits of AI in soccer are undeniable, there are also ethical concerns that need to be addressed:
- Fairness and Competition:The introduction of AI-powered players could create an uneven playing field, potentially leading to a dominance by teams with access to advanced AI technology. This could raise questions about fairness and the integrity of competition.
- Human Element and Emotional Connection:Soccer is a sport that evokes strong emotions and a sense of human connection. The presence of AI players, with their calculated and emotionless approach, could potentially diminish the emotional resonance and fan engagement that are central to the sport’s appeal.
- Job Displacement:The use of AI players could potentially displace human players, leading to unemployment and economic hardship in the sports industry. This raises concerns about the potential impact on the livelihoods of professional athletes and coaches.
AI-Assisted Coaching and Training in Soccer
Beyond the potential for AI players, the application of AI in coaching and training offers significant opportunities to enhance the performance of human athletes:
- Personalized Training Plans:AI algorithms can analyze player data, such as fitness levels, strengths, and weaknesses, to create personalized training plans that optimize individual development.
- Real-Time Performance Analysis:AI-powered tools can provide real-time feedback on player performance during training and matches, allowing coaches to make adjustments and optimize strategy based on data-driven insights.
- Injury Prevention and Rehabilitation:AI can help predict and prevent injuries by analyzing player movement patterns and identifying potential risks. It can also assist in developing personalized rehabilitation programs to help players recover from injuries more effectively.
Hypothetical Scenario: AI Players Competing Against Human Players
Imagine a future where a team of AI-powered soccer players, trained on years of simulated matches and equipped with advanced algorithms, takes on a team of human professionals. The AI players, with their superhuman reflexes, calculated passes, and strategic formations, dominate the field.
They move with a precision that leaves human players struggling to keep up. While the human team fights valiantly, the AI team ultimately prevails, showcasing the potential of AI to transform the sport.
Technological Advancements in AI: Deepmind Ai Learns To Play Soccer Using Decades Of Match Simulations
DeepMind’s soccer-playing AI, trained on decades of match simulations, showcases remarkable advancements in AI technology. The project demonstrates the power of simulation-based learning and its potential to revolutionize various fields beyond sports.
AI Technologies Employed
DeepMind utilized several cutting-edge AI technologies in their soccer project, including:
- Reinforcement Learning:The AI agent learned to play soccer by interacting with a simulated environment. It received rewards for positive actions, such as scoring goals, and penalties for negative actions, such as losing the ball. This process of trial and error, guided by rewards and penalties, enabled the AI to learn optimal strategies for playing soccer.
- Deep Neural Networks:The AI agent’s decision-making process was powered by deep neural networks, which are complex algorithms inspired by the structure of the human brain. These networks allowed the AI to process large amounts of data, identify patterns, and make predictions about the game’s future state.
- Simulation:DeepMind’s soccer project heavily relied on simulations to train the AI agent. This approach allowed the AI to play millions of games against itself, learning from its successes and failures in a controlled environment. The ability to run simulations at a rapid pace accelerated the learning process and enabled the AI to explore a vast range of strategic possibilities.
Advancements in AI Learning and Decision-Making
This project demonstrates significant advancements in AI learning and decision-making:
- Rapid Learning:The AI agent was able to learn complex strategies and adapt to different game situations within a relatively short time frame. This rapid learning capability is attributed to the use of simulation-based training and the power of deep neural networks.
- Strategic Decision-Making:The AI agent displayed impressive strategic thinking and decision-making abilities. It could anticipate opponent movements, plan attacks, and make tactical adjustments based on real-time game situations. This level of strategic awareness highlights the AI’s ability to understand and react to complex environments.
- Generalization:The AI agent was able to generalize its learning from the simulation to real-world scenarios. It could apply its knowledge and skills to different game situations and adapt to new opponents, showcasing the AI’s ability to transfer learning across various contexts.
Potential Applications Beyond Soccer, Deepmind ai learns to play soccer using decades of match simulations
The technologies employed in DeepMind’s soccer project have broad applications beyond sports:
- Robotics:The ability to learn and adapt quickly through simulation can be applied to develop more intelligent robots for various tasks, such as manufacturing, logistics, and healthcare.
- Autonomous Vehicles:AI algorithms trained on simulations can be used to improve the navigation and decision-making abilities of self-driving cars, enabling them to navigate complex environments and respond to unexpected situations.
- Drug Discovery:AI can be used to accelerate the drug discovery process by simulating the interactions between molecules and predicting the effectiveness of new drugs. This could lead to the development of more effective treatments for various diseases.
- Climate Modeling:AI can be used to improve climate models by analyzing vast amounts of data and simulating the effects of climate change. This could help us understand the impact of climate change and develop more effective mitigation strategies.
Examples of Other AI Projects Utilizing Similar Technologies
Several other AI projects utilize similar technologies to those employed in DeepMind’s soccer project:
- AlphaGo:DeepMind’s AlphaGo program, which defeated a professional Go player in 2016, also used deep neural networks and reinforcement learning to learn and play the game. AlphaGo’s success demonstrated the potential of AI to master complex games that were previously thought to be beyond the reach of computers.
- OpenAI Five:OpenAI’s Five Dota 2 bots, which defeated a professional Dota 2 team in 2019, also used reinforcement learning and deep neural networks to learn and play the game. This project showcased the ability of AI to master complex real-time strategy games with multiple players.
- Boston Dynamics’ Robots:Boston Dynamics, a robotics company, uses reinforcement learning and simulation to train its robots to perform complex tasks, such as walking, running, and jumping. This approach has led to the development of highly agile and versatile robots that can operate in challenging environments.