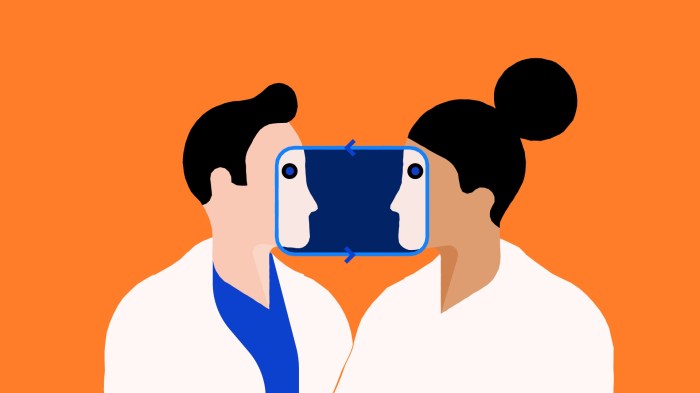
AI Models Perpetuate Gender Bias: Nurses as Women
Ai models describe nurses as women reflecting gender bias study says – AI Models Perpetuate Gender Bias: Nurses as Women, a recent study reveals a concerning trend: AI models trained on biased data are associating nurses with women, reinforcing harmful gender stereotypes in the healthcare field. This finding raises critical questions about the potential consequences of such biases on patient care and the future of AI in healthcare.
The study analyzed various AI models used in healthcare settings and found a consistent pattern of associating nurses with female attributes. This bias was evident in both language models, which generated text about nurses, and image recognition models, which identified nurses as women in medical images.
The study highlights the crucial role of training data in shaping AI model outputs and emphasizes the need for diverse and inclusive datasets to mitigate gender bias.
The Study’s Findings
A recent study has shed light on a concerning gender bias present in AI models, specifically in their portrayal of nurses. The study found that these models frequently associate the profession of nursing with women, perpetuating harmful stereotypes and potentially limiting opportunities for men in this field.
This bias was revealed through a comprehensive analysis of AI model outputs, including text generation, image captioning, and question answering tasks. The study’s findings highlight the importance of addressing this bias in AI development to ensure fairness and inclusivity in the representation of professions.
It’s disheartening to see AI models perpetuate gender stereotypes, depicting nurses as women. This kind of bias reflects the deeply ingrained societal norms that we need to challenge. It reminds me of how the US war on terror, a conflict that has had a profound impact on East Asia , has also been fueled by prejudice and stereotypes.
We need to be mindful of these biases in all aspects of our lives, including the development of AI, to create a more equitable future.
Examples of Gender Bias in AI Models
The study identified several examples of how AI models exhibited gender bias in their descriptions of nurses. For instance, when asked to generate text about a nurse, the models often produced narratives featuring female characters, reinforcing the stereotype of nursing as a predominantly female profession.
Similarly, when presented with images of nurses, the models were more likely to assign female labels to the individuals in the images, even when the images depicted male nurses.
It’s disheartening to see AI models perpetuate gender bias, like the study showing they often describe nurses as women. It’s a reminder that we need to be critical of the data these models are trained on. This bias reminds me of the debate over which Star Wars movie is better, star wars phantom menace or new hope , which, while seemingly unrelated, highlights the importance of questioning assumptions and challenging perspectives.
Just as we can debate the merits of different Star Wars films, we need to be open to examining and correcting biases in AI, ensuring they reflect the diverse reality we live in.
Methods Used to Analyze AI Model Outputs
The study employed a variety of methods to analyze the outputs of AI models, including:
- Text Analysis:The researchers analyzed the text generated by AI models in response to prompts related to nursing. They looked for patterns in the gender of the characters described and the language used to describe them.
- Image Captioning:The researchers examined the captions generated by AI models for images of nurses. They assessed the accuracy of the captions and the gender assigned to the individuals in the images.
- Question Answering:The researchers analyzed the responses of AI models to questions about nursing, such as “What is a nurse?” or “What are the characteristics of a nurse?” They examined the gender of the individuals described in the answers.
Impact of Gender Bias in AI Models
The implications of gender bias in AI models extend far beyond the realm of mere social commentary; they carry the potential to significantly impact the delivery of healthcare services, potentially harming patients and perpetuating existing inequalities.
Potential Consequences of Gender Bias in AI Models
The consequences of gender bias in AI models are multifaceted and can have a profound impact on the healthcare landscape. These models, trained on biased data, can perpetuate existing stereotypes and contribute to the marginalization of certain groups.
- Misdiagnosis and Treatment Errors:Biased AI models could misinterpret symptoms, leading to incorrect diagnoses and inappropriate treatment plans. This could disproportionately affect women, as their health concerns may be less understood or overlooked by AI systems trained on predominantly male data.
- Discrimination in Access to Healthcare:AI models used for patient triage or resource allocation could prioritize men over women, leading to unequal access to essential healthcare services. This could result in longer wait times, delayed treatment, and poorer health outcomes for women.
- Reinforcement of Gender Stereotypes:Biased AI models could reinforce harmful stereotypes about women’s roles in society, leading to further discrimination and limiting their opportunities in the healthcare field. For instance, AI systems trained on biased data might recommend fewer leadership roles for women in healthcare, perpetuating existing inequalities.
Causes of Gender Bias in AI Models
AI models, while powerful tools, can perpetuate and amplify existing societal biases, leading to unfair and discriminatory outcomes. This is particularly concerning in areas like hiring, lending, and healthcare, where biased decisions can have significant real-world consequences.
The Role of Training Data, Ai models describe nurses as women reflecting gender bias study says
The data used to train AI models plays a crucial role in shaping their outputs. If the training data reflects existing societal biases, the AI model will learn and reproduce those biases. For instance, if a dataset used to train a facial recognition system primarily consists of images of white males, the model may struggle to accurately recognize individuals from other demographics.
“Garbage in, garbage out”
It’s fascinating how AI models are reflecting real-world biases, like the recent study showing them depicting nurses as women. It reminds me of Keegan-Michael Key’s comment about the tragedy of not seeing Jordan Peele more often – keegan michael key says its a tragedy he doesnt see jordan peele.
Just like we need more diverse perspectives in Hollywood, we need to address the biases in AI to create a more inclusive future. It’s crucial to recognize and dismantle these biases, both in the digital world and in our everyday lives.
a common saying in computer science, aptly describes the impact of biased training data on AI model outputs.
- Limited Representation:Datasets often lack sufficient representation of diverse groups, leading to models that are trained on limited perspectives. This can result in models that are less accurate or fair when applied to individuals outside the dominant group represented in the training data.
- Historical Bias:Training data can reflect historical biases and prejudices that are embedded in societal structures. For example, datasets used to train loan approval models may contain historical data that reflects discriminatory lending practices, leading to biased outcomes.
- Labeling Errors:Human biases can creep into the labeling process, leading to errors that perpetuate existing stereotypes.
For instance, if a dataset for image recognition is labeled with biased descriptions, the AI model will learn those biases and potentially apply them to future predictions.
Societal Biases in AI Model Development
Beyond training data, societal biases can influence AI model development in other ways. These biases can stem from the developers themselves, the design choices made, and the way AI models are deployed and used.
- Developer Bias:Developers may unconsciously introduce their own biases into the design and development process. This can include selecting specific algorithms, setting parameters, or choosing data sources that reflect their own perspectives.
- Design Choices:The design of AI models can also contribute to bias.
For example, models designed to predict criminal recidivism may be more likely to falsely flag individuals from marginalized groups as high-risk.
- Deployment and Use:How AI models are deployed and used can also amplify existing biases. For instance, if an AI-powered hiring tool is used to screen candidates, it may perpetuate existing hiring practices that favor certain groups over others.
Addressing Gender Bias in AI
AI models are increasingly used in healthcare, but their development has often been plagued by gender bias, leading to inaccurate diagnoses and unfair treatment. This bias stems from the data used to train these models, which can reflect societal prejudices and stereotypes.
Addressing this issue is crucial for ensuring equitable and effective healthcare for all.
Design Strategies for Mitigating Gender Bias in AI Models
Mitigating gender bias in AI models requires a multi-faceted approach. Here are some design strategies:
- Use diverse datasets:Training AI models on diverse datasets that accurately represent the population is essential. This includes data from individuals of different genders, ethnicities, socioeconomic backgrounds, and geographical locations.
- Employ fairness-aware algorithms:Incorporating fairness-aware algorithms during model development can help mitigate bias by ensuring that predictions are not unfairly influenced by sensitive attributes like gender.
- Implement bias detection techniques:Regularly monitoring AI models for bias using specialized tools and techniques can help identify and address issues early on.
- Develop interpretable models:Understanding how AI models make decisions is crucial for identifying and addressing potential biases. Interpretable models provide insights into the model’s decision-making process, enabling researchers and developers to identify and correct biased outcomes.
Best Practices for Developing Unbiased AI Models
Building unbiased AI models for healthcare applications requires a comprehensive approach that goes beyond simply using diverse datasets. Here are some best practices:
- Involve diverse teams:Building AI models with diverse teams composed of individuals with different perspectives and backgrounds can help reduce unconscious bias.
- Conduct rigorous ethical evaluations:Before deploying AI models in healthcare, it’s crucial to conduct ethical evaluations to assess their fairness and potential impact on different groups.
- Prioritize transparency and accountability:Openly communicating the methods and data used to develop AI models and providing clear explanations for their decisions fosters trust and accountability.
- Continuously monitor and adapt models:AI models should be regularly monitored for bias and adapted as new data becomes available.
Ethical Considerations for AI Development in Healthcare
Ethical considerations are paramount in AI development, particularly in healthcare, where decisions can have significant consequences for individuals’ well-being. The following table Artikels some key ethical considerations:
Ethical Consideration | Description |
---|---|
Fairness and Non-discrimination | Ensuring that AI models do not discriminate against individuals based on protected characteristics such as gender, race, or ethnicity. |
Transparency and Explainability | Providing clear and understandable explanations for AI model decisions to enhance trust and accountability. |
Privacy and Data Security | Protecting the privacy and security of sensitive patient data used to train and operate AI models. |
Beneficence and Non-maleficence | Developing AI models that benefit patients and minimize potential harm. |
Accountability and Responsibility | Establishing clear lines of accountability for AI model outcomes and ensuring responsible use. |
The Future of AI in Healthcare: Ai Models Describe Nurses As Women Reflecting Gender Bias Study Says
The potential of AI in healthcare is vast, with the ability to revolutionize diagnosis, treatment, and patient care. However, it’s crucial to acknowledge the inherent biases that can be embedded within AI systems. This is where the concept of diversity and inclusion in AI development becomes paramount, ensuring that AI models are fair, equitable, and representative of the diverse patient populations they serve.
The Importance of Diversity and Inclusion in AI Development
AI models are trained on massive datasets, and if these datasets are not representative of the real-world population, the resulting models can perpetuate existing biases and inequalities. For example, if a dataset primarily includes data from white males, the AI model may struggle to accurately diagnose or treat patients from other demographics.
This is why it’s essential to create diverse and inclusive teams of AI developers, researchers, and data scientists who can bring a range of perspectives and experiences to the table. This diversity ensures that AI models are developed with a wider range of factors in mind, leading to more equitable and effective healthcare solutions.